The Digital Confluence: How Businesses Are Mastering Omnichannel Customer Engagement
In an era where the average American checks their phone 96 times a day, businesses are faced with a critical challenge: how to effectively engage customers across multiple digital channels. Enter the world of omnichannel customer engagement, where three seemingly outdated technologies—Bulk SMS, USSD, and IVR—are experiencing a renaissance, working in concert to create seamless customer experiences.
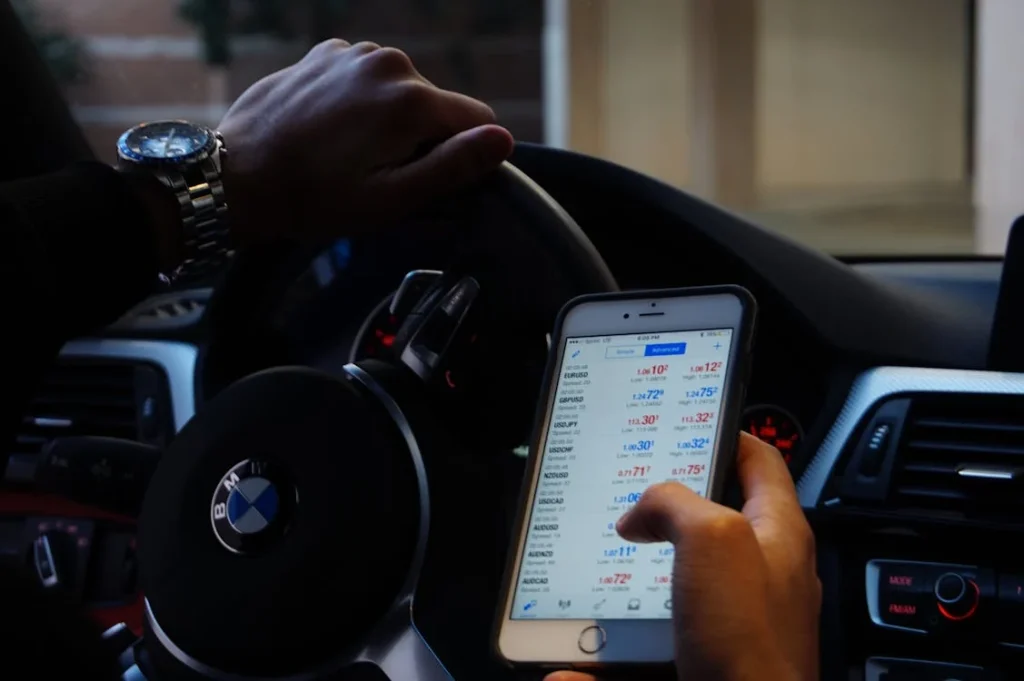
The Unlikely Trio: SMS, USSD, and IVR
At first glance, these technologies might seem like relics of a bygone digital age:
- Bulk SMS (Short Message Service): The humble text message, limited to 160 characters.
- USSD (Unstructured Supplementary Service Data): A protocol used by mobile phones for communicating with service providers’ computers.
- IVR (Interactive Voice Response): Automated phone systems that interact with callers using voice and keypad inputs.
Yet, in the hands of innovative companies, these technologies are far from obsolete. They’re being integrated and supercharged to create powerful, inclusive, and surprisingly personal customer engagement strategies.
The Power of Integration
“It’s not about using the newest technology, it’s about using the right technology for your audience,” says Maria Rodriguez, Chief Customer Officer at OmniTech Solutions. “By integrating SMS, USSD, and IVR, we can reach customers where they are, with the devices they have, in ways that feel natural to them.”
This integration allows for a true omnichannel experience. A customer journey might start with a bulk SMS campaign, continue through a USSD menu for quick information retrieval, and culminate in an IVR call for more complex interactions—all seamlessly connected.
Real-World Impact
Banking on Inclusion
In Kenya, Equity Bank has revolutionized banking for the unbanked population by integrating these technologies. “Our customers can check balances via USSD, receive transaction alerts through SMS, and handle more complex queries through our IVR system,” explains John Mwangi, Equity Bank’s Head of Digital Banking. “This integration has allowed us to serve millions who might otherwise be excluded from traditional banking services.”
The impact is staggering: since implementing this integrated approach, Equity Bank has seen a 40% increase in rural customer engagement and a 25% reduction in branch visits, significantly reducing operational costs.
Retail Revolution
Target, the American retail giant, has also embraced this integrated approach. “We use bulk SMS for flash sale announcements, USSD for quick product checks in-store, and IVR for customer service,” says Emily Chen, Target’s VP of Customer Experience. “The key is the seamless transition between these channels. A customer can start their journey with an SMS, use USSD in-store, and if they need more help, they’re transferred to our IVR system with their context intact.”
This strategy has resulted in a 15% increase in customer satisfaction scores and a 10% boost in sales from SMS-promoted events.
The Inclusivity Factor
One of the most compelling aspects of this integrated approach is its inclusivity. “Not everyone has a smartphone or reliable internet access,” notes Dr. Alvin Chang, Professor of Digital Communications at MIT. “By leveraging these technologies, companies can engage with a broader demographic, including older populations and those in rural or developing areas.”
This inclusivity is not just good ethics—it’s good business. A study by the Pew Research Center found that 19% of Americans rely on smartphones for internet access, with higher percentages among lower-income and rural populations. For these groups, technologies like USSD and IVR can be lifelines to essential services.
Challenges and Concerns
Despite its potential, this integrated approach is not without challenges. Privacy concerns are at the forefront, with critics arguing that the extensive data collection required for effective omnichannel engagement could lead to misuse.
“There’s a fine line between personalization and invasion of privacy,” warns Eva Blanco, a digital rights activist. “Companies need to be transparent about data usage and give customers control over their information.”
Technical challenges also abound. Integrating legacy systems with modern databases and ensuring real-time data synchronization across channels can be complex and costly.
The Future of Omnichannel Engagement
As artificial intelligence and machine learning continue to advance, the potential for these integrated systems grows exponentially. Imagine an AI that can predict which channel a customer is likely to use next and preemptively prepare personalized responses.
“The future is not just omnichannel, but omniscient channel engagement,” predicts Raj Patel, CEO of FutureTech AI. “We’re moving towards systems that don’t just react to customer needs, but anticipate them across all available channels.”
A Balancing Act
As businesses rush to adopt these integrated strategies, they must balance efficiency with empathy, automation with authenticity. The goal is not to replace human interaction but to enhance it—to use technology to make customer engagement more personal, not less.
“At the end of the day, customers want to feel heard and valued,” reminds Rodriguez. “Our job is to use these technologies in a way that amplifies the human touch, not diminishes it.”
In a world where digital fragmentation is the norm, the integration of Bulk SMS, USSD, and IVR offers a path to unified, inclusive, and effective customer engagement. It’s a reminder that sometimes, the most powerful innovations come not from inventing new technologies, but from reimagining and integrating the ones we already have.
As businesses navigate this digital confluence, one thing is clear: the future of customer engagement lies not in the channels themselves, but in the seamless, thoughtful, and human-centric integration of them all.